A few weeks ago, I was out with friends watching a football game. We had a great time. For all of you who love watching a football match with friends, the best moment is after the game, where you stay to discuss the outcome and analyze what happened. We were all caught up in the excitement of the match, especially when our team didn’t secure the win.
Some of us felt they played exceptionally well, creating numerous scoring opportunities. Others argued that the opposing team’s defense was outstanding, making it challenging for our team to break through. Others thought we were just unlucky. As the discussion unfolded, we realized our biases as supporters or coaches might be clouding our judgment. We wonder if there was a way to objectively measure our team’s performance. That’s when looking at the xG stats, particularly the Expected Goals (xG) data, can give a clearer perspective.
Disclosure: Please note that some of the links below area affiliate links and at no additional cost to you I will earn a commission. Know that i only recommend products services and brands i have personally used and stand behind. When you use one of my affiliate links, the company compensates me, which helps me run this blog and keep my in -depth content free of change for readers (like you). Read our disclosure for more info.
Table of Contents
We all talk about Expected Goals but We Do not Know it
Analyzing team performance is crucial in sports, especially in football where the outcomes can be decided on small margins. One of the most insightful metrics used in modern football analysis is Expected Goals (xG). But what exactly is xG, and how can it help us understand a team’s performance and the expected points for the season??
Understanding this xG Statistic has been a fascinating process for me. Getting familiar with this metric not only deepens my appreciation for the details of football analysis but also offers invaluable insights into team dynamics and player performances. It has provided me with the opportunity to further embrace my love for football and understand why it’s often hailed as the “King of Sports“. Moreover, it has show me how small details can have a significant impact on the outcome of a game, a tournament, or even a league title race. Additionally, it has increase my admiration for the exceptional abilities possessed by certain players, whether it be their ability in scoring goals or their skills for preventing them.
Expected Assists is a similar metric that coaches and analysts are using to predict the expected outcome from a specific situation but this is something we can see in another article. Expect from a coaching and analysis toll, xG stats is also a fan engagement way for a team to show to their supporters their performance levels.
Understanding the xG
Expected Goals is a statistical metric that estimates the likelihood of a goal being scored from a particular shot. Is a metric that evaluates the chance quality. This number considers various factors such as the type of shot, the shot location, the angle, the opposition’s positioning, the distance from goal, and the situation leading up to the shot. Essentially, xGgoals gives us a way to quantify the quality of scoring chances.
The calculation of Expected Goals involves analyzing thousands of shots from past games to determine the probability of each shot being an actual goal. This probability is then assigned a value between 0 and 1. For instance, a tap-in from close range might have an xG value of 0.9, indicating a 90% big chance of scoring, while a shot from outside the box might have an Expected Goals value of 0.1.
The greatest teams in the world are recruiting Data Analysts to calculate these numbers. Bayern Munich, Manchester United, Real Madrid from La liga, Manchester City, and many others have people who analyze thousands of data points to extract significant results that will help their teams and players perform better at the highest level. The increase in matches per year and the need to compete in the local championship, as well as in the Champions League, Europa League, and Conference League, has increased the need to collect and analyze information. In addition to team performance, players like Robert Lewandowski, the World Cup winner Lionel Messi, and Harry Kane need to analyze their individual performance and quantify their finishing skill..
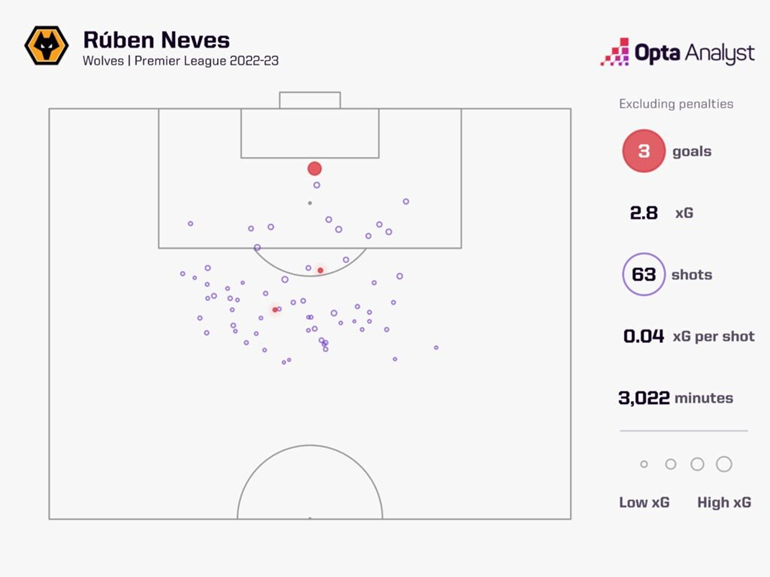
Source: Opta
Consider a scenario where a striker, receives a through ball inside the penalty area. The forward, known for his clinical finishing, finds himself in a favorable position with free space and a clear sight of the goal. As he prepares to shoot, the Excepted Goals model assesses various factors: the distance from goal, the angle of the shot, the type of shot (e.g., a low-driven shot or a chip), the presence of defenders, and the goalkeeper positioning.
Based on similar shots from past situations, the Expected Goals model assigns a value to this scoring opportunity. Suppose the value assigned to the player’s shot is 0.7. This means there is a 70% probability that the player will score from this position, considering all relevant factors.
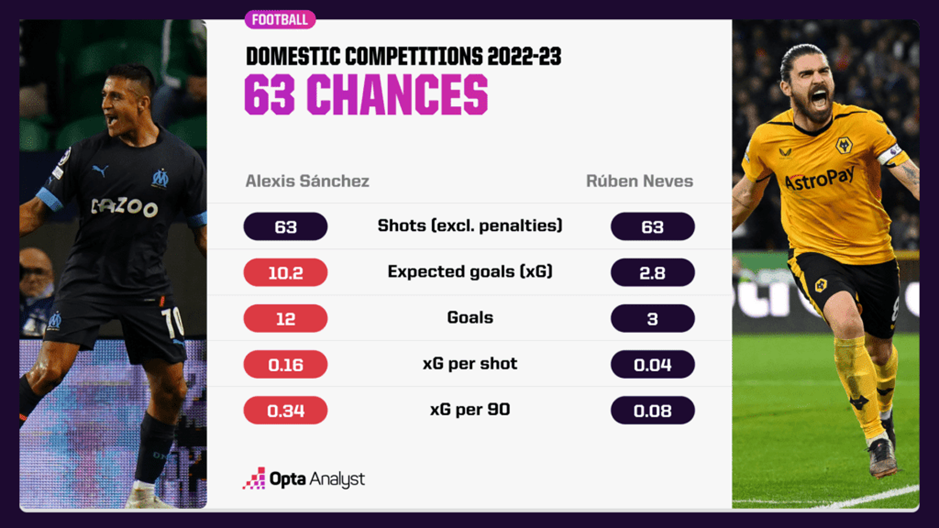
Source: Opta
Let’s take, for example, the Premier League season 2023 – 2024 season table. Manchester City secured the Premier League title by scoring 96 goals and conceding 34. Their relative xG metrics were 89.55 for and 37.27 against. These numbers reveal that Man City scored 6.45 goals more than expected and conceded 3.27 fewer goals than expected. A positive difference between Expected Goals and actual goals indicates that the team has efficient forwards who can convert low-probability chances into goals, as well as strong goalkeepers or defenders capable of dealing with dangerous situations.
This observation reflects the reality of Manchester City’s performance. Erling Haaland’s proficiency in scoring goals from challenging positions may account for the variance between actual goals and xG. Examining the data from the preceding four seasons (2019 – 2023), we observe that the league-winning team consistently demonstrated a positive difference between xG and actual goals.
Team | Season | Goals | xG | Difference |
Manchester City | 2022 – 23 | 94 | 84.32 | +9.68 |
Manchester City | 2021 – 22 | 99 | 93.40 | +5.60 |
Manchester City | 2020 – 21 | 83 | 77.72 | +5.28 |
Liverpool | 2019 – 20 | 85 | 75.19 | +9.21 |
So, one evident conclusion drawn from this data analysis is that teams require players who can convert scoring opportunities from challenging positions and situations, along with defenders capable of preventing easy goals. While this may seem obvious, the numbers confirm its significance. Simply scoring goals in line with a team’s performance isn’t sufficient for winning the league; they must exceed expectations. They need to outperform.
Now, let’s examine another example of a team that underperformed. In the season 2023 – 24, Liverpool showcased exceptional performance in creating goal-scoring opportunities. They possessed talented players and implemented good team strategies, resulting in numerous high-quality chances. Their xGs value stood at an impressive 94.79, suggesting that the team should have scored nearly 95 goals. To simplify, if Liverpool had capitalized on the clear chances they created, they would have been strong contenders for the title. In a league setting, an additional 9 goals could potentially translate to 7 – 9 more points.
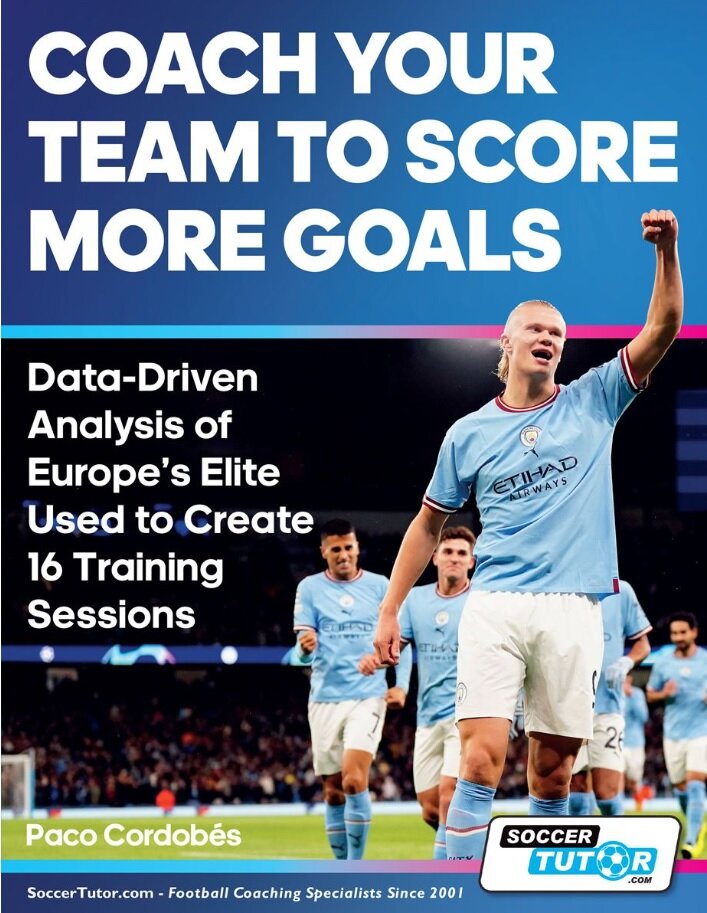
Data-Driven Analysis of Europe’s Elite Used to Create 16 Training Sessions
- Data-Driven Analysis
- Match Examples
- Training Sessions
- Score More Goals!
Another way to use the xGs is describe a player’s ability to score goals. Usually the forwards at the end of the league they will score the goals that the xG is showing. A team when is recruiting a forward, should not just see the numbers he scored in one season. It is important to compare the number with the xGoals as to identify is a player had a season out of the normal.
For example, if a forward scores 20 goals but the xG is 14.95 it means that he was overperforming. His actual ability is approximately 15 goals based on his awareness to position inside the area and his timing to arrive for the chance is lower. The possibility is that the next season will score less than 20 goals.
Heung-Min Son had an impressive season in 2020-21, scoring 17 goals despite having an Expected Goals number of just 11.63. Many of his goals came from individual actions and situations where the opponent’s positioning was good and there was a low probability of conversion. However, Son’s exceptional ability allowed him to outperform his xG. In the following season, Son scored 23 goals from an xG of 16.71. While it’s undeniable that Son possesses the skill to score challenging goals, the question arises: can a team rely solely on a player’s ability to consistently overperform?
In the 2022-2023 season, Son scored 10 goals with an xG of 8.6, and in the subsequent season, he scored 17 goals with an xG of 15.98. Although he consistently surpassed his xG, his goals did not exceed 20, which is considered the norm for elite goal scorers. Therefore, based on these numbers, a team could evaluate Son as a player who typically scores between 14 to 18 goals per season. If this goal-scoring range aligns with a team’s requirements, they may choose to proceed with Son. However, if a team prioritizes a player who consistently produces over 20 goals per season, Son may not be their first choice.
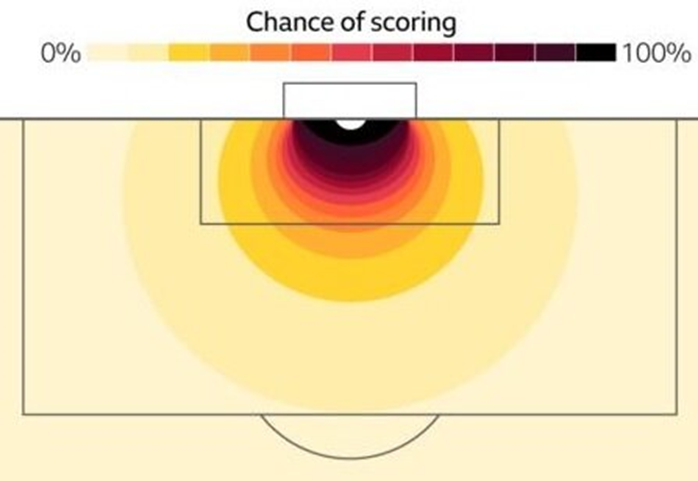
Why Analyze Team Performance Based on Expected Goals?
- Objective Measurement: Using Expected Goals provides an objective measurement of a team’s performance, excluding biases and subjective interpretations. Unlike traditional statistics like goals scored, which can be influenced by factors such as exceptional individual performances or random chance, xG focuses on the quality of scoring opportunities. This objective assessment allows teams to evaluate their performance accurately, irrespective of the final scoreline.
- Identifying Strengths and Weaknesses: Expected Goals analysis helps in identifying specific strengths and weaknesses within a team’s gameplay. By “reading” the chance quality of chances created and conceded, teams can pinpoint areas for improvement. For instance, if a team consistently generates high xG but struggles to convert these chances into goals, it indicates a deficiency in finishing skill, decision-making in the final third or quality of the center forward. Similarly, if a team concedes high xG against them, it highlights defensive vulnerabilities that need addressing.
- Improving Team Strategy: Incorporating Expected Goals into performance analysis enables teams to develop more effective strategies. Coaches and analysts can leverage xG data to fine-tune offensive and defensive tactics. For instance, if analysis reveals that a team is more successful in scoring from crosses than through balls, the coaching staff can adjust their gameplay to capitalize on this strength. Moreover, understanding xG can help teams devise defensive strategies to limit opponents’ high-quality scoring opportunities, thereby improving overall defensive solidity.
Why Expected Goals Matter
The importance of xG lies in its ability to provide a deeper understanding of a team’s performance. It measures how effective a team is in creating and converting scoring opportunities, and helps in comparing actual performance to expected performance. xG allows us to see whether a team is underperforming or overperforming.
If a team has a high Expected Goals but scores few goals, it suggests they are creating good chances but failing to convert them. Conversely, a team scoring more goals than their Expected Goals suggests might be relying on luck or exceptional finishing. By comparing a team’s actual goals scored to their xG, analysts can identify whether a team’s results are sustainable. A team consistently outperforming their xG may be over-reliant on factors that are not consistently replicable, such as individual brilliance or opponent errors.
Collecting and Analyzing xG Data
Reliable Expected Goals data can be obtained from various sources such as sports analytics companies, football databases, and even some advanced sports media outlets. Several tools and software are available for xG analysis, including Opta, StatsBomb, Stats Perform and Wyscout that provide detailed xG metrics and visualizations. xG can highlight how well a team is creating scoring opportunities. By analyzing xG values over multiple games, one can determine if a team’s attack is consistently generating high-quality chances. Defensively, xG can be used to assess the quality of chances a team is conceding. A team with a low xG against them is typically good at preventing high-quality shots from their opponents and has great reaction choosing the correct defensive action.
Match Game and Scouting
Coaches can use xG to develop offensive strategies that focus on creating high Expected Goals chances. This involves improving the quality of passes, shot selection, and positioning. They can choose the area on the pitch they would like to move the ball and make an assist or a shot. Defensively, understanding xG can help in devising strategies to limit opponents to low xG shots, which are less likely to result in goals.
Also, the scouting and player’s recruiting departments can use specific metrics to evaluate the true ability of a player to score goals or the true ability of a goalkeeper to save shots.
Limitations and Advanced Techniques
While Expected Goals is a powerful tool, it’s not infallible. It may not account for every details of a game, such as player skill level, weather conditions, psychological factors or even a lucky day. Factors like defensive pressure, player fatigue, or game context can influence shot quality but might not be fully captured by xG models. xG Chain and xG Build-Up are advanced metrics that consider the contribution of all players involved in the buildup to a shot, providing a more comprehensive view of team play. xG can also be applied to goalkeepers, assessing the quality of shots they face and their save performance relative to the xG of those shots.
Conclusion
Expected Goals (xG) has revolutionized football analysis, offering a deeper, more nuanced understanding of team performance. By assessing the quality of scoring chances, xG helps teams improve their strategies, track their progress, and make data-driven decisions. As football analytics continues to evolve, xG will undoubtedly remain a key tool for teams, analysts, and fans alike.
FAQs
- What are Expected Goals (xG)?
Expected Goals (xG) is a metric that estimates the probability of a goal being scored from a particular shot based on various factors.
- How accurate is the xG metric?
xG is generally accurate but not perfect. It provides a good indication of shot quality but may not capture all situational factors.
- Can xG be used for individual player analysis?
Yes, xG can be applied to individual players to assess their shooting efficiency and the quality of chances they create.
- How do teams implement xG data in their strategies?
Teams use xG data to develop offensive and defensive strategies, focusing on creating high-quality chances and limiting opponent opportunities.
- Where can I find reliable xG data?
Reliable xG data can be found from sports analytics companies, football databases, and advanced sports media outlets. Examples are Opta, Statsbomb and Wyscout.